Generative AI represents an excellent, transformative leap in artificial intelligence (AI). This advanced technology is known for its ability to generate text, images, and other media that resemble human creation. The attention these Gen AI tools and technologies are garnering from across industries is nothing short of remarkable.
This trend is specifically driven by its advanced cognitive capabilities and context-aware outputs. However, the leaders are also being cognitive about its challenges, specifically concerning data security, ethical considerations, and operational integrity. This blog explores further aspects of Generative AI in quality engineering, its key risks, and mitigation strategies.
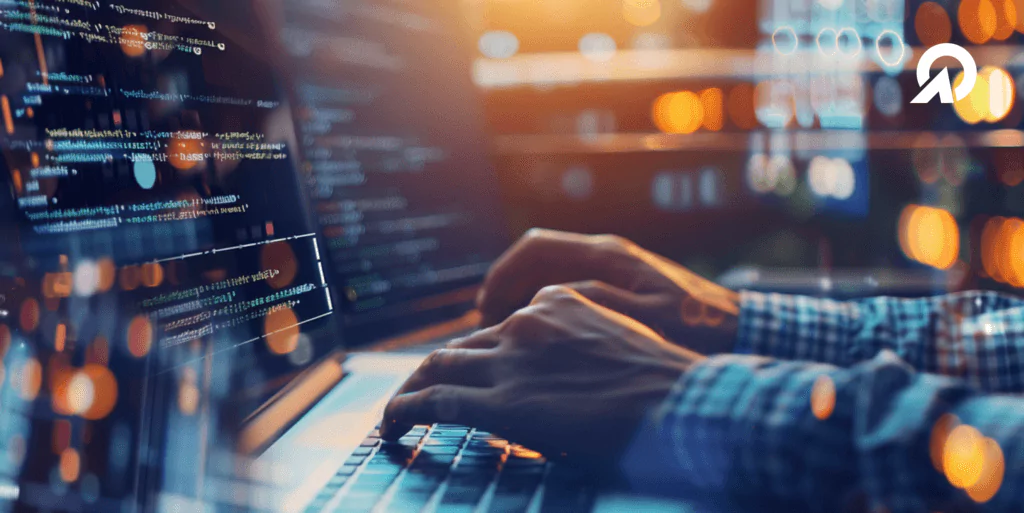
Gen AI’s Contribution to Quality Engineering
Quality engineering aims to ensure that all products or services manufactured within a particular industry or sector meet customer requirements. It must be made in a way that exceeds explicitly their expectations.
Quality engineering includes all activities which assist in improving the quality of the product and the production process while identifying and reducing waste. Quality Engineering methods and tools are often implemented via a cross-functional approach that involves multiple businesses and engineering disciplines.
Gen AI is a perfect fit for all quality engineering services and solutions because it surpasses traditional artificial intelligence capabilities. This becomes possible by empowering machines to comprehend information and create, imagine, and innovate efficient products.
A recent study confirms Generative AI’s status as a breakthrough technology, with 43% of 1,405 surveyed enterprises investing in it. GenAI technology brings immense value to software testing lifecycle in enhancing test coverage, expediting test cycles, boosting tester productivity, and thereby delivering high-quality software products.
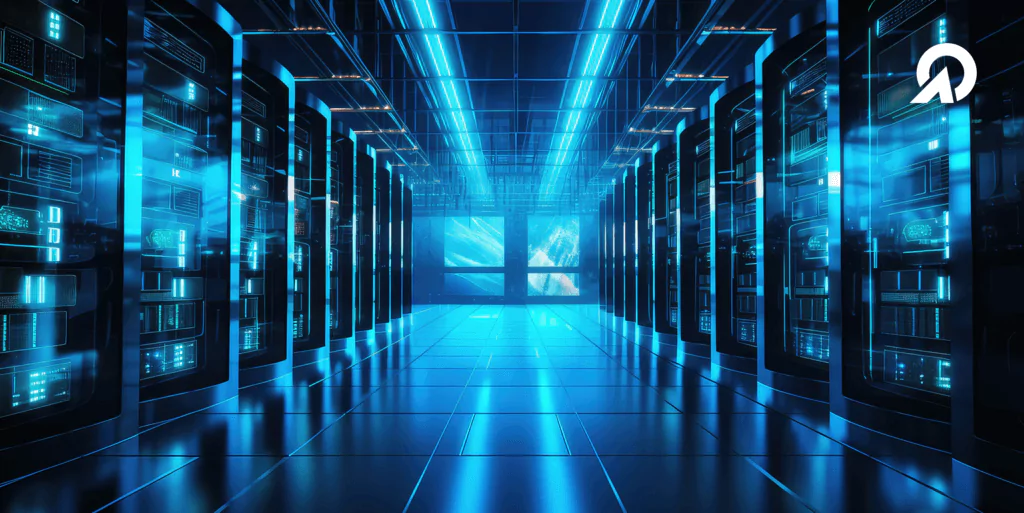
Top Generative AI Risks and Strategies for Mitigation
The transformative impact of Gen AI on quality engineering is still evolving. However, organizations striving to maintain a competitive advantage must incorporate GenAI in their operations sooner rather than later. At the same time, they must also address the following key risks:
1. Output Quality Issues
Generative AI can misclassify defects, leading to unreliable test results. False positives mark non-issues as defects, wasting time on unnecessary debugging. False negatives, on the other hand, let critical defects slip through, increasing the risk of production failures. These inaccuracies stem from incomplete training data, inconsistent model performance, or an over-reliance on AI-generated outputs.
Mitigation Strategies:
- Hybrid Testing Approach: Combine AI-driven testing with human validation to verify results.
- Threshold Tuning: Fine-tune anomaly detection models to reduce false classifications.
- Data Refinement: Continuously improve training datasets for better defect classification.
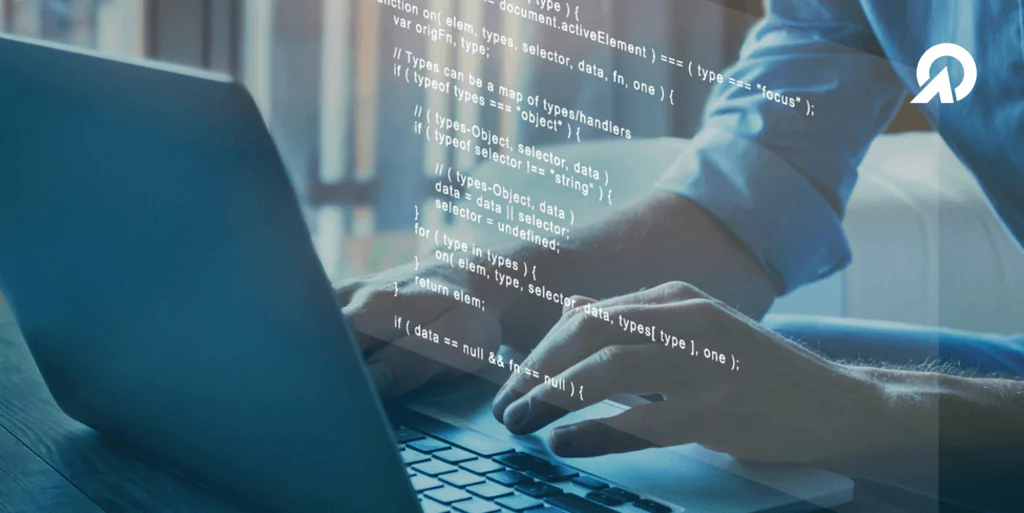
2. Lack of Explainability and Traceability
AI models often function as black boxes, making it difficult to understand why certain test cases are generated or why specific defects are flagged. Without clear explanations, debugging AI-driven test failures becomes complex, and teams may struggle to justify test results to stakeholders.
Mitigation Strategies:
- Explainable AI (XAI): Use AI models that provide insights into how they classify test cases.
- Version-Controlled Test Reports: Maintain logs of AI-generated decisions and results.
- Human-in-the-Loop Validation: Ensure critical test cases undergo manual review.
3. Not Suitable to Resolve Every Issue
Generative AI can accelerate testing, but it is not a universal solution for all testing challenges. AI models may struggle with subjective quality assessments, such as usability, accessibility, and user experience testing, etc. These aspects require human intuition, empathy, and domain expertise—elements that AI cannot replicate as of now.
Additionally, AI-driven testing lacks the ability to understand business context deeply. It may generate extensive test cases but fail to recognize mission-critical workflows, compliance regulations, or industry-specific risks. Relying solely on AI could lead to gaps in validation, ultimately affecting software reliability and user satisfaction.
Mitigation Strategies:
- Use AI Selectively: Apply AI for test generation, defect prediction, and optimization, but rely on human testers for subjective assessments.
- Domain-Specific Testing: Ensure periodically validate business-critical scenarios that AI may overlook with human in loop approach.
- Augment AI with SME Insights: Collaborate with domain experts to refine AI-generated outcomes.
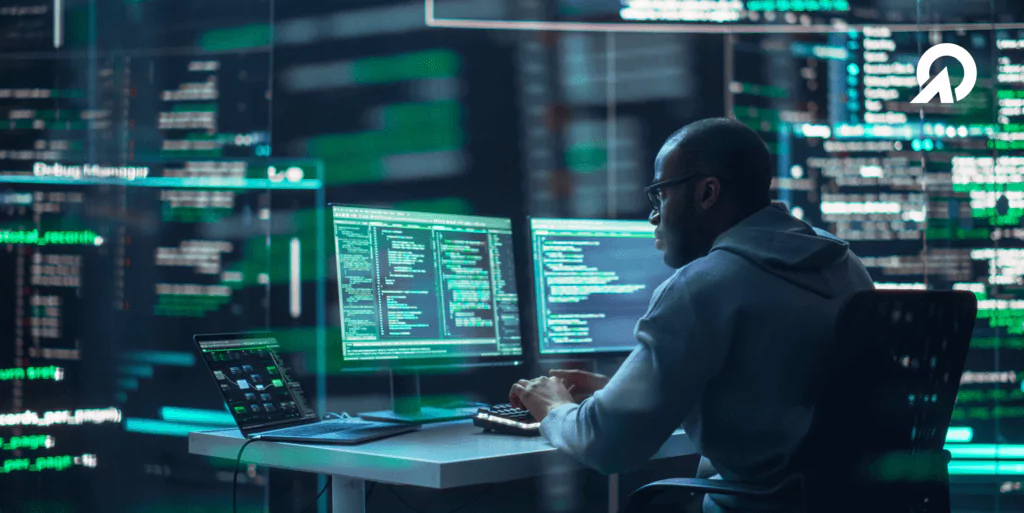
4. Data Privacy and Cybersecurity Risks
AI models require large datasets to learn and improve, but they may inadvertently store or expose sensitive information. If an AI system is trained on production data without adequate safeguards, it could introduce compliance risks, particularly in industries governed by regulations such as Banking, Healthcare, Insurance, etc. Organizations using AI-driven test automation must ensure that their models do not leak personally identifiable information (PII) or sensitive enterprise data.
Another major security risk is AI model poisoning, where adversaries manipulate training data to introduce vulnerabilities. Attackers could inject malicious code into AI-generated scripts, leading to security breaches. Without robust monitoring, AI-based automation might unknowingly introduce security loopholes into production environments.
Mitigation Strategies:
- Data Masking: Remove or anonymize sensitive information before training AI models.
- Federated Learning: Train AI models on decentralized data sources to enhance security.
- Regular Security Audits: Continuously evaluate AI-generated test data for vulnerabilities.
- AI Model Monitoring: Detect and prevent adversarial attacks targeting AI-based test automation.
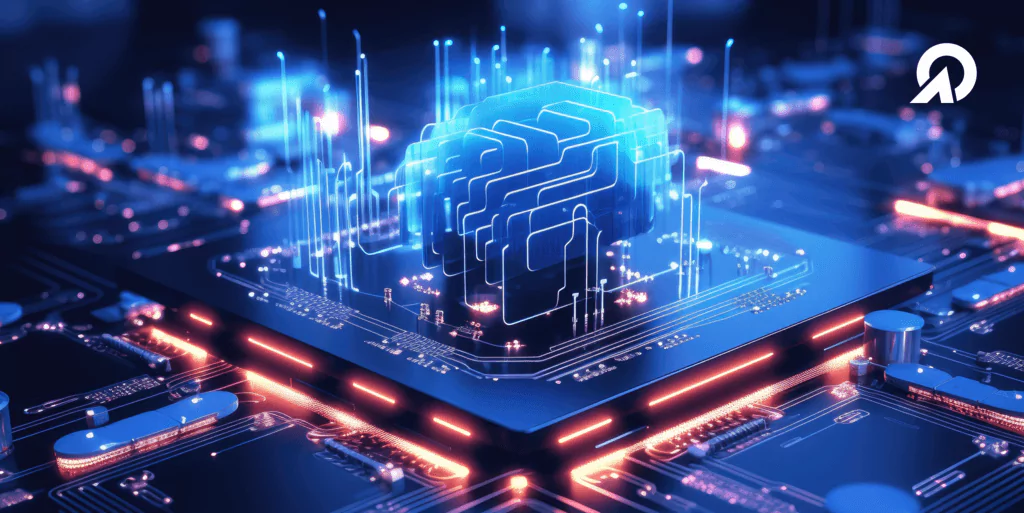
5. Bias in Generated Output
AI models learn from historical data, which may introduce inherent biases. If an AI-driven testing system is trained on datasets that only include specific device configurations, OS versions, or user demographics, it may generate test cases that overlook diverse scenarios. This can result in poor test coverage, accessibility gaps, and a lack of inclusivity in software quality validation.
Bias can also emerge in defect classification, where AI models prioritize commonly occurring issues while neglecting edge cases that impact real-world users. If left unchecked, this can create blind spots in software testing, reducing overall product reliability.
Mitigation Strategies:
- Diverse Training Datasets: Incorporate real-world test scenarios to reduce bias.
- Model Retraining: Regularly update AI models to adapt to changing software requirements.
- Ethical Framework: Establish ethical framework and guidelines to proactively measure and validate the generated outcomes.
6. Over-Reliance on AI
AI-driven testing improves efficiency, but excessive dependence on AI can introduce risks. If AI-generated test cases, scripts or user stories lack domain expertise, they may fail to capture business-critical test scenarios. Additionally, AI alone cannot replace the nuanced judgment of experienced testers. Context-aware, usability, and exploratory testing, etc. still require human intelligence.
Another risk of over-reliance on AI is “automation debt,” where teams blindly trust AI-generated scripts/test cases without regular reviews. As applications evolve, AI-created test cases may become outdated, leading to inefficient test execution and misleading results.
Mitigation Strategies:
- Balanced Approach: Combine AI-driven testing with expert validation.
- Periodic Test Reviews: Manually inspect AI-generated test results for relevance.
- AI as an Assistant: Use AI to optimize testing efforts rather than completely automate them.
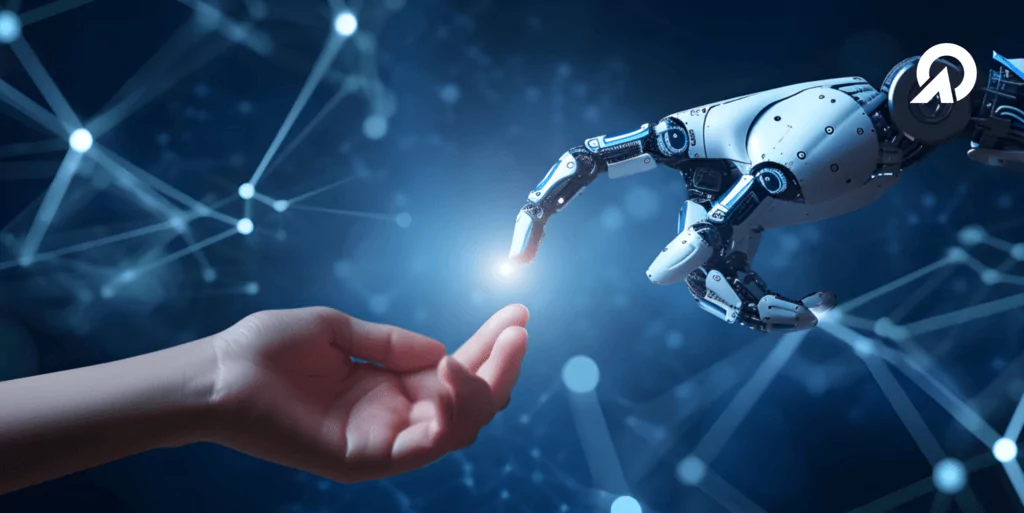
Best Practices for Reducing Risk Associated with AI in Quality Engineering
To effectively integrate AI into Quality Engineering while minimizing risks, organizations should adopt these best practices:
- Blend AI with Traditional Testing: AI should enhance—not replace—human testing efforts. Human oversight ensures critical test scenarios are not overlooked.
- Continuously Monitor AI Performance: Regularly track AI-generated test cases and adjust models to maintain accuracy and relevance.
- Implement Ethical AI Guidelines: Establish governance policies for responsible AI use, ensuring fairness, transparency, and security.
- Use AI for Test Optimization: Focus on AI-driven insights for prioritizing tests and predicting defects rather than full automation.
- Train QE Teams on AI Integration: Equip testers with knowledge on AI capabilities, limitations, and best practices to maximize its benefits.
Generative AI is transforming Quality Engineering, making test automation smarter, defect prediction sharper, and efficiency greater. But with these advancements come challenges—false positives, biased test scenarios, security risks, and the danger of over-relying on AI-driven decisions. To truly harness AI’s potential, organizations must adopt a balanced, risk-aware approach—leveraging AI where it excels while maintaining human oversight for quality, fairness, and security.
This is where QMentisAI, QualiZeal’s latest GenAI-powered product, comes into play. Designed specifically for Quality Engineering, QMentisAI eliminates AI-driven biases, enhances defect detection accuracy, and optimizes test coverage with intelligent recommendations. It empowers teams with context-aware insights, self-learning capabilities, and real-time analytics, ensuring AI-driven testing remains trustworthy, efficient, and risk-free.
By implementing best practices and proactive mitigation strategies, QE teams can unlock the full potential of AI-driven testing without compromising software quality.
Are you ready to embrace AI in your testing strategy? Connect with QualiZeal to explore AI-powered QE solutions! 🚀